Watch all the Transform 2020 sessions on-demand here.
This week, VentureBeat held its annual VB Summit 2018, an intimate gathering of business executives to discuss the role AI can play in transforming their business.
Throughout boardroom sessions and onstage interviews, one issue companies repeatedly raised is difficulty finding data scientists. No surprise there. This shortage has been well-documented for some time now, as competition for machine learning experts and talent to develop neural nets has resulted in salaries comparable to those of professional athletes.
But there’s more to it than that. Another idea frequently repeated at VB Summit was that transforming to a data-driven business requires change from the wider organization, not just the machine learning experts themselves.
Though some may believe trying to explain ML to business executives is a waste of time, CI&T chief digital evangelist Lucas Persona believes the biggest breakthroughs belong to companies that enable data science teams to work alongside other employees.
June 5th: The AI Audit in NYC
Join us next week in NYC to engage with top executive leaders, delving into strategies for auditing AI models to ensure fairness, optimal performance, and ethical compliance across diverse organizations. Secure your attendance for this exclusive invite-only event.
Working shoulder-to-shoulder with the rest of the company means a data science team should not be treated like the wizard behind the curtain or philosophers in an ivory tower.
“ML has become in many organizations more and more … siloed. We are the ML team, the DS team, and every time somebody comes to talk to us, we will say these crazy words [and] they will feel bad about themselves and leave, so it’s kind of off-putting,” he said.
This breakdown in communication only widens the divide between machine learning experts and the rest of the company.
Instead, the entire organization should be encouraged to become AI-literate, so that everyone can think about ways to tackle the company’s biggest problems. The more teams work together, the more other parts of the company may understand the limitations that can be placed on machine learning, and the more other divisions can explain to the data science team the kinds of challenges that could be encountered when retrieving data or implementing an ML model.
Companies that hire data scientists without attempting to cross-pollinate between other departments can create solutions that go underutilized or sit on a shelf, Persona said. But once the whole company is thinking about ML, initiatives can move beyond creating models based on datasets that already exist to creating datasets needed to solve a company’s most pressing problems.
“What is best? For us to spend six months on the data we have for a problem that doesn’t really solve a lot of things or spending six months figuring out how to acquire the data — because once we do it, then we can solve a problem, then we can tackle the biggest questions there,” he said. “That’s pretty much like you opening your fridge and saying ‘Let’s cook tonight’ and being limited to what you have in the fridge. The other approach is ‘OK, what’s the best dinner I can make tonight?’ and then I go buy the ingredients I need.”
The takeaway from these discussions was that the trend of paying data scientists exorbitant amounts of money for their expertise isn’t going anywhere, but there’s more to building an AI-first company than hiring the latest talent out of MIT or Carnegie Mellon. It’s also about preparing the rest of your team to work alongside them.
For AI coverage, send news tips to Kyle Wiggers and Khari Johnson — and be sure to bookmark our AI Channel.
Thanks for reading,
Khari Johnson
AI Staff Writer
P.S. Please enjoy this video of Gong, winner of the AI Innovation showcase at VB Summit.
From VB
Pick a color and this AI system will craft a logo
Researchers at Maastricht University have developed an AI system that can generate logos from 12 different color keywords.
Amazon says Alexa is an ESFJ (Updated)
Alexa is an ESFJ on the Myers-Briggs personality type indicator, according to an Amazon VP. Personality is a part of Alexa forging connections with people.
How SETI uses off-the-shelf AI to search for extraterrestrial life
SETI is using off-the-shelf machine learning technology from Nvidia and Google to search for other forms of life in the universe.
Aramark exec: ‘Trying to explain AI and machine learning to the C-suite is a waste of time’
AI and machine learning are still not well understood by most C-level executives, a group of experts discussed at VB Summit 2018.
DARPA wants to build ‘contextual’ AI that understands the world
The Defense Advanced Research Projects Agency (DARPA) is a pioneer in AI. At VB Summit 2018, Dr. Brian Pierce, director of its Innovation Office, spoke about ongoing efforts.
Bright Machines raises $179 million for factory automation with robots
Bright Machines wants to make it easier to quickly manufacture hardware through the use of machine learning and robotics.
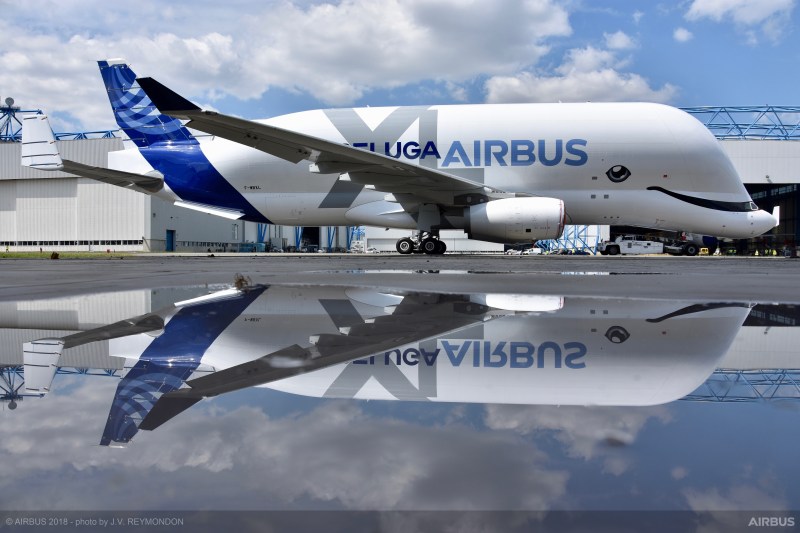
Above: Airbus BelugaXL
Airbus believes its AI can eliminate flight delays
Airbus’ VP of artificial intelligence spoke at VB Summit 2018 about the innovative ways the aircraft manufacturer is employing machine learning.
Beyond VB
The first piece of AI-generated art to come to auction
AI artwork sells for $432,500 — nearly 45 times its high estimate — as Christie’s becomes the first auction house to offer a work of art created by an algorithm. (via Christie’s Auction)
No, machine learning is not just glorified statistics
The sentiment that machine learning is really nothing to get excited about, or that it’s just a redressing of age-old statistical techniques, is growing increasingly ubiquitous; the trouble is it isn’t true. (via Towards data science)
Look to Africa to advance artificial intelligence
If AI is to improve lives and reduce inequalities, we must build expertise beyond the present-day centres of innovation, says Moustapha Cisse. (via Nature)
Google ponders the shortcomings of machine learning
Scientists of AI at Google’s Google Brain and DeepMind units acknowledge machine learning is falling short of human cognition and propose that using models of networks might be a way to find relations between things that allow computers to generalize more broadly about the world. (via ZDNet)