testsetset
Cassie Kozyrkov has served in various technical roles at Google over the past five years, but she now holds the somewhat curious position of “chief decision scientist.” Decision science sits at the intersection of data and behavioral science and involves statistics, machine learning, psychology, economics, and more.
In effect, this means Kozyrkov helps Google push a positive AI agenda — or, at the very least, convince people that AI isn’t as bad as the headlines claim.
Anxiety
“Robots are stealing our jobs,” “AI is humanity’s greatest existential threat,” and similar proclamations have abounded for a while, but over the past few years such fears have become more pronounced. Conversational AI assistants now live in our homes, cars and trucks are pretty much able to drive themselves, machines can beat humans at computer games, and even the creative arts are not immune to the AI onslaught. On the flip side, we’re also told that boring and repetitive jobs could become a thing of the past.
People are understandably anxious and confused about their future in an automated world. But, according to Kozyrkov, artificial intelligence is merely an extension of what humans have been striving for since our inception.
June 5th: The AI Audit in NYC
Join us next week in NYC to engage with top executive leaders, delving into strategies for auditing AI models to ensure fairness, optimal performance, and ethical compliance across diverse organizations. Secure your attendance for this exclusive invite-only event.
“Humanity’s story is the story of automation,” said Kozyrkov, speaking at the London AI Summit this week. “Humanity’s entire story is about doing things better — from that first moment that someone picked up a rock and banged another rock with it because things could get done faster. We are a tool-making species; we rebel against drudgery.”
The underlying fear that AI is dangerous because it can do things better than humans doesn’t hold water for Kozyrkov, who argues that all tools are better than humans. Barbers use scissors to cut hair because clawing it out with their hands would be a less-than-desirable experience. Gutenberg’s printing press enabled the mass production of texts at a scale that would have been impossible for humans with pens to replicate. And pens themselves opened up a whole world of opportunity.
“All of our tools are better than human — that’s the point of a tool,” Kozyrkov continued. “If you can do it better without the tool, why use the tool? And if you’re worried about computers being cognitively better than you, let me remind you that your pen and paper are better than you at remembering things. My bucket is better than me at holding water, my calculator is better than me at multiplying six-digit numbers together. And AI is also going to be better at some things.”
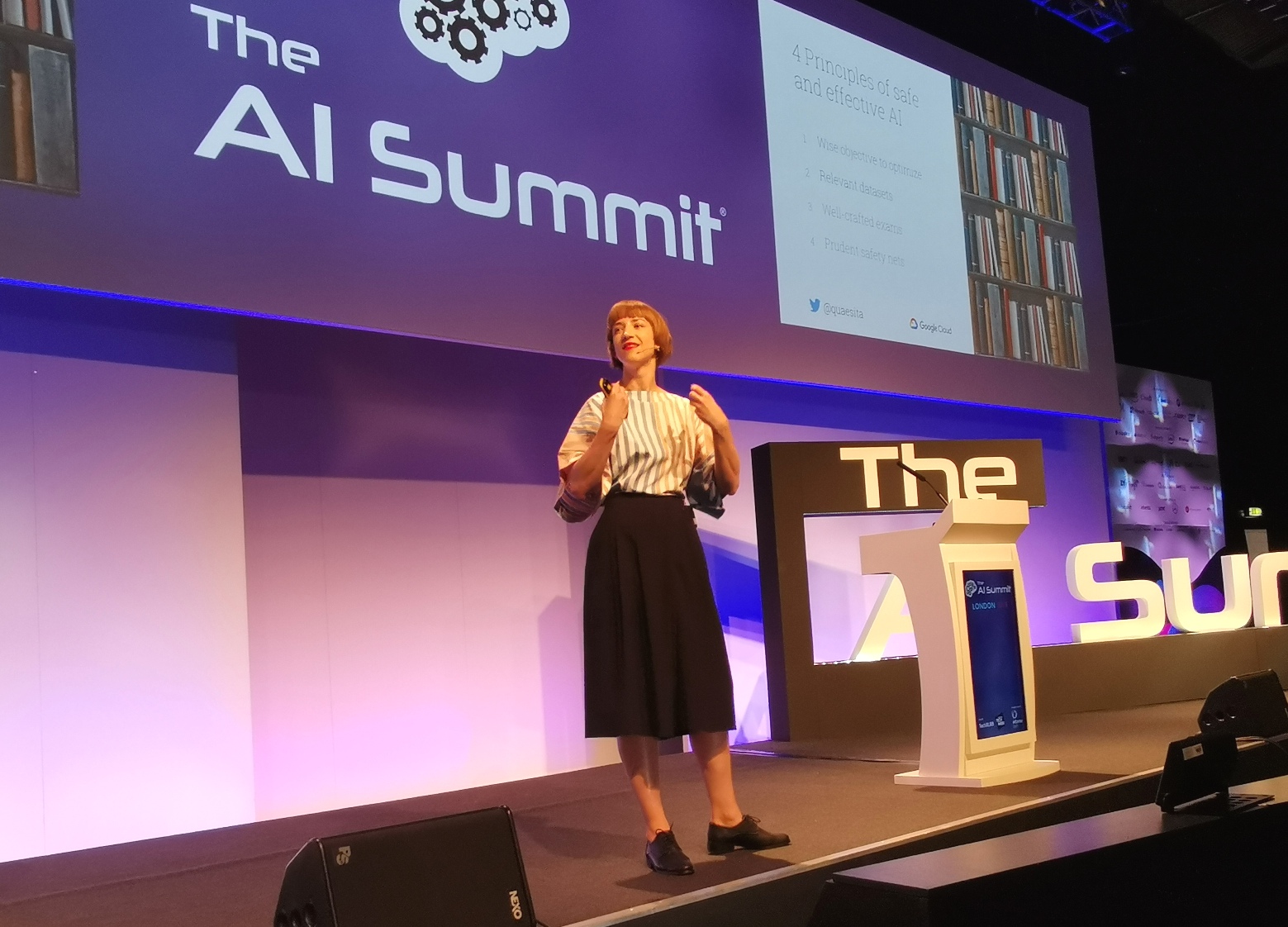
Above: Cassie Kozyrkov, “chief decision scientist” at Google, speaking at AI Summit (London) 2019
Of course, the underlying fear many hold in relation to AI and automation isn’t that it will be better at things than humans. For many, the real danger lies in the unbridled scale with which governments, corporations, and any ill-meaning entity could cast a dystopian shadow over us by tracking and micro-managing our every move — and achieving a clandestine grand vision with next to no effort.
Other concerns relate to factors such as algorithmic prejudices, a lack of sufficient oversight, and the ultimate doomsday scenario: What if something goes drastically — and unintentionally — wrong?
Biases
Researchers have already demonstrated the inherent biases in facial recognition systems such as Amazon’s Rekognition, and Democratic presidential candidate Senator Elizabeth Warren recently called on federal agencies to address questions around algorithmic bias, such as how the Federal Reserve deals with money lending discrimination.
But less attention is given to how AI can actually reduce existing human biases.
San Francisco has recently claimed it will use AI to reduce bias when charging people with crimes, for example, by automatically redacting certain information from police reports. In the recruitment realm, VC-backed Fetcher is setting out to help companies headhunt talent by leveraging AI, something it claims can also help minimize human prejudices. Fetcher automates the process of sourcing potential candidates by trawling online channels and uses keywords to determine skill sets an individual may have that are not listed on their profile. The company pitches its platform as an easy way to remove bias from recruitment, because if you train a system to follow a strict set of criteria focused purely on skills and experience, elements such as gender, race, or age won’t be taken into account.
“We believe we can leverage technology to address several forms of hiring bias to help companies build more diverse and inclusive organizations,” Fetcher cofounder and CEO Andres Blank told VentureBeat at the company’s relaunch last year.
But concerns about how AI could proliferate systemic discrimination are top of the agenda in many AI circles, with Microsoft urging the U.S. government to regulate facial recognition systems and researchers working on methods to reduce bias in AI without hampering the accuracy of predictive results.
The human element
The bottom line is that AI is in its relative infancy, and we’re still figuring out how to address issues such as algorithmic bias. But Kozyrkov said that biases demonstrated by AI are the same as existing human biases — data sets used to train machines are exactly like textbooks that are used to educate people.
“Data sets and textbooks both have human authors — they’re both collected according to instructions made by people,” she said. “One of them is easier to search than the other. One of them might be in paper format, the other electronic, but they’re pretty much the same thing. If you give your students a textbook that was written by a horribly prejudiced author — do you think your student is not going to pick up some of those same prejudices?”
In the real world, of course, well-regarded, peer-reviewed journals or textbooks should have sufficient oversight to combat any blatant prejudices or bias — but what if the author, their data sources, and the teacher who encourages their students to read the textbook all have the same prejudices? Any pitfalls may not be discovered until much later, by which point it’s too late to stop any ill effects.
Thus Kozyrkov believes “diversity of perspective” is imperative to ensuring minimal bias.
“The more different kinds of eyeballs that you have looking at your data and thinking about what the consequences of using these examples to express yourself would be, the more likely you are to catch those potentially bad cases,” she said. “So in AI, diversity is a must-have — not a nice-to-have. You do need to get those different perspectives looking and thinking about how using these examples might impact the world.”
Testing
Similar to the real world of student exams, testing AI algorithms and machine learning models before they’re deployed is key to ensuring that they’re capable of carrying out the tasks they are set.
A human student may perform extremely well in an exam situation if they’re asked the exact questions they studied beforehand, but that could be because they have a great memory rather than a complete understanding of the subject at hand. To test wider comprehension, students need to be given questions that allow them to apply what they have learned.
Machine learning operates on the same premise — there’s a modeling error known as “overfitting,” where a specific function is too closely aligned to training data, which can throw up false positives. “Computers have really good memory,” Kozyrkov noted. “So the way you actually test them is that you give them real stuff that’s new, that they couldn’t have memorized, that’s relevant to your problem. And if it works then, then it works.”
Kozyrkov drew parallels between four principles of safe and effective AI and four basic tenets of teaching human students, saying that you need:
- Wise teaching goals — think about what you want to teach your students.
- Relevant and diverse perspectives.
- Well-crafted tests.
- Safety nets.
The latter principle is particularly important, because it can be easy to overlook the “What if things go wrong?” scenario. Even the most well-crafted, well-intentioned AI system can fail or make mistakes — in fact, the better the system is, the more dangerous it can be in some ways, just like human students.
“Even if your student is really good, they could still make mistakes,” Kozyrkov said. “In fact, in some ways, a ‘C’ student is less dangerous than an A+ student, because with the ‘C’ student you’re used to them making mistakes, and so you already have a safety net in place. But [with] the A+ student, if you’ve never seen them make a mistake before, you might think they never make them. It just might take you a little longer, and then it’s a catastrophic failure.”
This “safety net” can take multiple forms, but it often involves building a separate system and not “over-trusting your ‘A+’ student,” as Kozyrkov put it. In one example, a homeowner configured his smart camera and lock system to activate if it spotted an unfamiliar face — but somewhat humorously, it falsely identified the homeowner as the Batman image on his T-shirt and refused him entry.
The “safety net” in this instance was the PIN on the lock, and the homeowner could also have used a function in his mobile app to override the AI.
All of this leads back to a point that may be obvious to many but perhaps bears repeating: AI is a reflection of its creators. Therefore, we need to focus on implementing systems and checks to ensure those building the machines (the “teachers”) are responsible and accountable.
There is a growing consensus around the importance of “machine teaching,” with the likes of Microsoft recently stating that the next frontier in AI will entail using human professionals’ expertise to train machine learning systems — regardless of the experts’ knowledge of AI or ability to code.
“It’s time for us to focus on machine teaching, not just machine learning,” Kozyrkov noted. “Don’t let the science fiction rhetoric distract you from your human responsibility and paying attention to the humans who are part of this all the way through from the beginning. From the objective set by leaders, those data sets authored by engineers [and] checked out by analysts and decision-makers, those tests that are done by statisticians, and those safety nets built by reliability engineers — all of that has a lot of the human component in it.”