Watch all the Transform 2020 sessions on-demand here.
In the U.S., about 12% of women will develop invasive breast cancer in their lifetime, and an estimated 268,000 of new cases are expected to be diagnosed by the year 2020 alone. Fortunately, the average five-year survival rate is quite high — 85-99%, according to the American Cancer Society — as is the average 10-year survival rate (83%). But as always, early detection is the difference maker.
To this end, in a paper published this month in the journal Radiology, scientists at IBM Research based in Haifa, Israel detail an AI model capable of predicting the development of malignant breast cancer in patients within a year. Peer-reviewed results show that their system correctly forecasts the development of 87% and 77% of cancerous and benign cases, respectively. Moreover, trained on a novel corpus of 9,611 mammograms and health records, it was able to identify breast cancer in 48% of people that otherwise wouldn’t have been flagged, at an accuracy comparable to radiologists (as defined by the American benchmark for screening digital mammography).
The Haifa team’s work builds on a study conducted by scientists at IBM’s Zurich office and the University of Zurich, which architected a system that can detect and classify tumor and immune cells as well as their relationships. Similar efforts to improve breast cancer screening accuracy are underway at Google, MIT, and NYU.
“Our model could one day help radiologists to confirm or deny positive breast cancer cases,” wrote Michal Chorev, IBM Research staff member and coauthor of the Radiology paper, in a blog post. “While false positives can cause an enormous amount of undue stress and anxiety, false negatives can often hamper how early a cancer is detected and subsequently treated.”
June 5th: The AI Audit in NYC
Join us next week in NYC to engage with top executive leaders, delving into strategies for auditing AI models to ensure fairness, optimal performance, and ethical compliance across diverse organizations. Secure your attendance for this exclusive invite-only event.
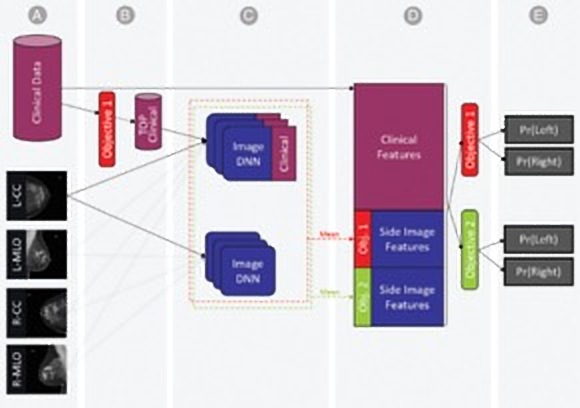
Above: The researchers’ breast cancer AI model.
To compile a training data set, Chorev and colleagues sourced deidentified mammography images linked to clinical data and biomarkers corresponding to patients’ electronic health records, including (but not limited to) thyroid function, reproductive history, white blood cell profiles, metabolic syndrome, and other information. They fed this data — which also included follow-ups from biopsies, cancer registry data, lab results, and codes for various other procedures and diagnoses — into a machine learning model that mapped connections among clinical risk factors to anticipate biopsy malignancy and differentiate normal from abnormal screening examinations.
When it came to mammography scans, the team principally used craniocaudal (CC) and mediolateral oblique (MLO) views — two standard views in mammography that are often compared in assessing lesions — from Israeli health care providers Maccabi Health Services and Assuta Medical Center. In the end, their data set contained 52,936 images from 13,234 women who underwent at least one mammogram between 2013 and 2017, and who had health records for at least one year prior to the mammogram.
An AI algorithm trained on mammograms for each prediction task and extracted the probabilities of these tasks, as well as those of imaging tasks for each view. Lastly, they concatenated the imaging features as well as the entire set of clinical features into a single representation of patients’ breasts. The final probability for either cancer-positive biopsy or normal/abnormal differentiation were estimated using a separate AI model.
The researchers say that their system sussed out clinical factors that might contribute to elevated risk but were not used in prior work, such as white blood cell profiles and thyroid function tests. “We plan to continue analyzing these clinical risk elements to better understand their impact and connections to an individual’s personalized risk,” added Chorev.