Watch all the Transform 2020 sessions on-demand here.
Designing conversational AI isn’t as Sysiphean as it sounds. Tools like Google’s Dialogflow, Microsoft’s Bot Framework, and Amazon’s Lex make it easier than it used to be, and the folks at Uber hope to eliminate any remaining barriers with a development platform all their own. It’s called Plato Research Dialog System, and it was released today on GitHub.
As the folks at Uber AI (Uber’s AI research division) explain in a lengthy blog post, Plato is designed for building, training, and deploying conversational AI agents to enable data scientists and hobbyists to collect data from prototypes and demonstration systems. It features a “clean” and “understandable” design, and it integrates with existing deep learning and model-tuning optimization frameworks that reduce the need to write code.
This first iteration of Plato (version 0.1) supports interactions through speech, text, or structured information (e.g., dialogue acts), and each conversational agent can interact with human users, other agents, or data. (Plato can spawn multiple agents and ensure that input and output data is passed to each agent appropriately, and keep track of the conversation.) Additionally, Plato can incorporate pretrained models for every conversational agent component, and each component can be trained during interactions or from data.
Plato accomplishes this with a modular design that breaks data processing into seven steps: speech recognition, language understanding, state tracking (aggregating information about what has been said and done so far), API calls (e.g., searching a database), dialogue policies (generating an abstract meaning of an agent’s response), language generation (converting said abstract meaning into text), and speech synthesis. Plato supports a range of conversational AI architectures, and each element can be trained using popular machine learning libraries such as Uber’s Ludwig, Google’s TensorFlow, and Facebook’s PyTorch.
June 5th: The AI Audit in NYC
Join us next week in NYC to engage with top executive leaders, delving into strategies for auditing AI models to ensure fairness, optimal performance, and ethical compliance across diverse organizations. Secure your attendance for this exclusive invite-only event.
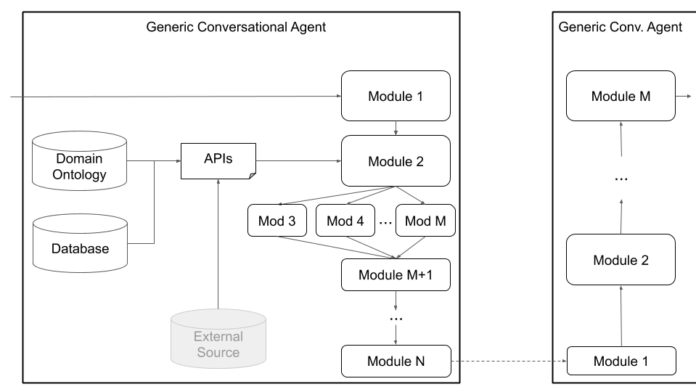
Above: Plato’s generic agent architecture supports a wide range of customization, including joint components, speech-to-speech components, and text-to-text components.
In a testament to its extensibility, Plato users can define their own architectures or plug their own components by providing a Python class name and package path to that module, as well as the model’s initialization arguments. So long as the modules are listed in the order they should be executed, Plato handles the rest, including wrapping the input and output, chaining and executing the modules (either serially or in parallel), and facilitating the dialogues.
When it comes to data logging, Plato keeps track of events in a structure called the Dialogue Episode Recorder, which contains information about previous dialogue states, actions taken, current dialogue states, and more. There’s even a custom field that can be used to track anything that doesn’t fall under the defined categories.
“We believe that Plato has the capability to more seamlessly train conversational agents across deep learning frameworks, from Ludwig and TensorFlow to PyTorch, Keras, and other open source projects, leading to improved conversational AI technologies across academic and industry applications,” wrote Uber AI researchers Alexandros Papangelis, Yi-Chia Wang, Mahdi Namazifar, and Chandra Khatri. “[We’ve] leverage[d] Plato to easily train a conversational agent how to ask for restaurant information and another agent how to provide such information; over time, their conversations become more and more natural.”
The release of Plato follows the debut of the aforementioned Ludwig, an open source “toolbox” built on top of Google’s TensorFlow framework that allows users to train and test AI models without having to write code. Last December, Uber brought Horovod, a framework for distributed training across multiple machines that its developers have used internally to support self-driving vehicles, fraud detection, and trip forecasting, in open source to the LF Deep Learning Foundation.