The mobility revolution may have the potential to transform cities, but in the short term the rise in ride-hailing apps, bike sharing, and electric scooters is giving many local officials fits. A healthy dose of data and machine learning may help get this movement back on track.
That’s the bet that San Francisco-based StreetLight Data is making. The company is helping cities harness the explosion of data being generated by everything from smart city sensors to mobile phones to new transportation modes, in a bid to reinvent urban planning. As cities groan under rising populations and pollution, making more effective use of data could be the key to making them habitable over the long run.
“We are taking advantage of the massive amounts of mobile devices that are roaming around,” said Laura Schewel, CEO and cofounder of StreetLight Data. “The lack of insight into fundamental transportation patterns is inhibiting our ability as a society to make smart decisions about really important things. Like, how to allocate trillions of dollars of infrastructure budget every year, or how to place electric vehicle chargers in an efficient way.”
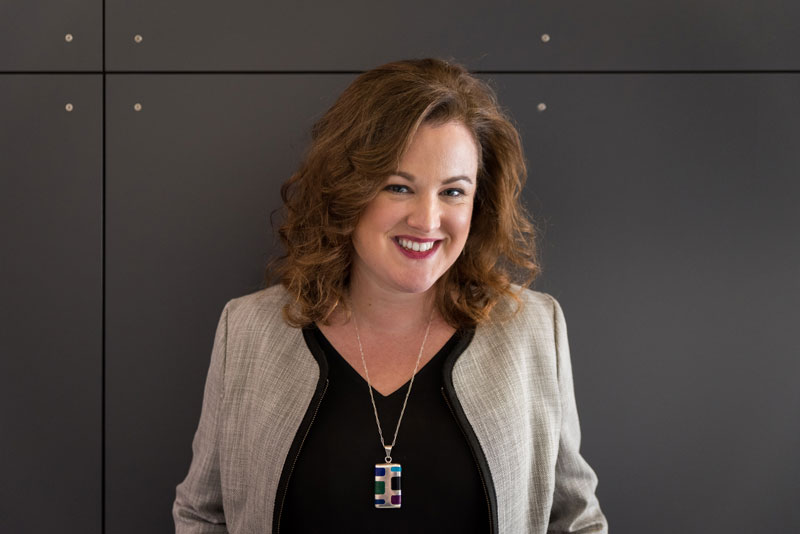
Above: Laura Schewel, CEO and cofounder of StreetLight Data
Founded in 2012, StreetLight is part of a broader global movement that is using new digital tools to gain greater insight into how people move and the way transportation functions. The company has raised a total of $29 million in venture capital, including most recently a $10 million round in 2018.
June 5th: The AI Audit in NYC
Join us next week in NYC to engage with top executive leaders, delving into strategies for auditing AI models to ensure fairness, optimal performance, and ethical compliance across diverse organizations. Secure your attendance for this exclusive invite-only event.
The company’s platform draws on trillions of GPS and cellular data points from numerous sources, including cell phones, sensors placed around smart cities, connected cars, and internet of things deployments. Almost anything that transmits information about location can be plugged into the company’s service, which anonymizes the information it receives via partnerships even as it allows for extremely granular tracking.
The data is pulled into StreetLight’s machine learning environment, where it can be processed for rapid insights into traffic patterns for cars, trucks, bikes, scooters, and pedestrians. The company’s machine learning helps urban planners identify whether data is coming from a bike, car, or someone walking down the street. Based on that information, they can model how and where investments in infrastructure could improve circulation.
“If you have a limited pool of funds to build cycling lanes, where should they go?” Schewel said. “Well, it depends on what do you want. If what you want is to facilitate bike safety for low-income people, then certain places are your top priority: the places where low-income people take long bike trips. And we can show you where that’s true. But if your priority is to get as many vehicles off the road as possible, then let’s find the places where most people are driving two miles per trip, because that’s silly.”
Typically, StreetLight is a neutral player and clients are accessing the data without the company necessarily knowing about the scope of projects. But in a more recent case, the company did get directly involved in a way that illustrates how the service works.
Data-driven decisions
The city of Santa Clara and Siemens were awarded grant money from the California Energy Commission to create a blueprint for placing electric vehicle charging stations. The idea was to take a more holistic, proactive planning approach rather than approving charging stations one at a time as private companies proposed them.
While Santa Clara and Siemens were doing community outreach, StreetLight’s role was to help formulate the range of answerable questions that can be used to drive decision making from the data.
“We worked a little more hand-in-hand because we designed a special dashboard to support EV charging in a way that really worked for Santa Clara and Siemens’ goals,” Schewel said.
The city, for instance, wanted to know where it could place EV stations that might be subsidized with local dollars in a place that would most likely benefit local residents or delivery drivers for local businesses. But how one defines those goals can quickly create a lot of contradictions.
“It turns out people wanted a lot of different things,” Schewel said. “They wanted the charger to be in a place where lots of people park, but then they also want the charger to be in a place where lots of local trucks park, and the people are local, and it’s not just in residential areas for people who have driveways. And it has to be in a place where utilities are OK with charging happening. And basically, there’s no point where all of those things are true.”
The key for Santa Clara will be ranking the different priorities and giving them different weights, questions that ultimately go to a community’s values and politics, and which data alone cannot solve. Once that happens, planners can simply click on different weights and rankings for those priorities to see how they shift the placement of EV charging stations.
“Santa Clara is saying, ‘If you care about these six things, here’s the map,'” Schewel said. “But if you give more weight to thing number three, here’s how the map changes. And that allows an iterative conversation that is data-driven about the trade-offs of different priorities.”
Future planning
Such capabilities could create a sea change in how cities plan, as well as in the profession of urban planning. Schewel, whose background is urban planning, said times are different for people with similar training.
“When I started grad school, transportation engineering was not what creative, innovative people did,” she said. “And now, applications for urban planning master schools are shooting up. In the past decade it’s become so clear that this field is so important and so critical.”
This trend is driven by the impact that such digital tools make possible, which relieve planners of the often long and tedious process of gathering data that can still be quite limited in scope. “The city departments and planners we work with have been waiting for something like this,” Schewel said. “We’re unleashing behavior for clients, especially those that get our monthly subscriptions, where they start running insane amounts of projects because they are just so excited to understand what’s happening on the roads.”
At the same time, the mobility market is creating intense competition for such talent, which could pose problems for the public sector.
“The other thing we’re seeing, and we are a little part of that now, is that people with transportation planning are being recruited by Uber, and scooter companies are recruiting them, and I’m recruiting them,” Schewel said. “All the cities have to compete, and frankly we pay more.”
This talent will be essential because the trends driving this shift will only accelerate in the coming years. Planners are already looking ahead to how best to adapt to autonomous vehicles, even if the timeline for such technology remains fuzzy. The prospect of robotic delivery vehicles constantly roaming the streets and sidewalks is likely to throw another wrinkle into planning decisions.
And recently, StreetLight began working with Uber to discuss planning around aerial transportation. Such modes, whether it’s delivery drones or autonomous vehicles carrying passengers, have the potential to replace longer road trips across a region, which could in turn change traffic flows and priorities for certain public transportation systems.
At the same time, the richness of data is also expanding. With more and more devices like scooters and connected bikes on the streets, StreetLight is accumulating even more specific data covering wider geographic ranges. More wearables are adding yet another layer of information.
And as 5G rolls out, Schewel said, the company is expecting that it will enable unprecedented amounts of location information, with much greater accuracy.
“We’ve been operating for decades in a data void,” Schewel said. “So I really am excited about these flexible tools that are part of enabling the community and the policymakers to be their own data scientists.”