Watch all the Transform 2020 sessions on-demand here.
Like Google, Uber, and countless other startups and tech giants, Lyft is developing cars it hopes will someday ferry passengers sans driver — a task that’s easier said than done. In a Medium post published this morning, Lyft’s Level 5 team — the division devoted to autonomous vehicle (AV) research and development — laid out a few of the challenges its engineers have encountered to date, while pulling back the curtains on solutions and general progress.
Picture this: A car driving down a freeway encounters another car cutting across multiple lanes to make an exit. Preventing a collision would require the first car to slow down, but just how dramatic should that slowdown be? Lyft’s self-driving prototypes tap what the company calls a “human-inspired” planning approach to determine this. Originally, they used a baseline AI model that didn’t account for the velocity of obstacles, but the newest model naturally learned from human driving to marginally slow down for cars performing high-velocity cut-ins.
“Ultimately, this blended approach resulted in a more comfortable and natural ride that was tuned to human preferences,” wrote Lyft’s Level 5 team. “We believe using a combination of rule-based systems, learning-based systems, and human driving data will result in an overall system-level solution that exhibits the best of all worlds.”
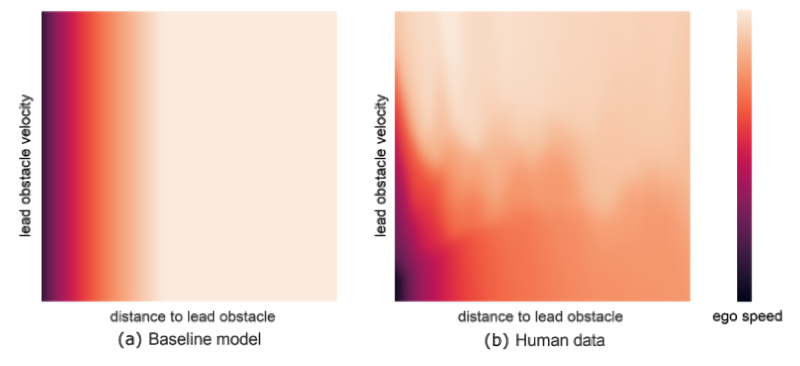
Above: A comparison between the approaches advocated by Lyft’s planning models.
Level 5 engineers say they took inspiration from Maslow’s hierarchy of needs and Asimov’s three laws of robotics in architecting the AV decision-making system. At the base of their pyramidal model is safety and legality — Lyft vehicles validate that planned behaviors are safe and in compliance with local laws before executing them. Although the blog post doesn’t elaborate, the safety bit is likely akin to Nvidia’s Safety Force Field and Intel subsidiary Mobileye’s Responsibility-Sensitive Safety (RSS), both of which prevent crashes with mathematical calculations backed by physics-based verifications.
June 5th: The AI Audit in NYC
Join us next week in NYC to engage with top executive leaders, delving into strategies for auditing AI models to ensure fairness, optimal performance, and ethical compliance across diverse organizations. Secure your attendance for this exclusive invite-only event.
Next, the planning model considers the notion of perceived safety, which here refers to minimizing passengers’ and other drivers’ perceptions of being unsafe, even when actual safety isn’t at significant risk. (In practice, this might entail increasing the distance to a lead car or ensuring the autonomous car doesn’t get too close to a lane divider.) The penultimate tier is comfort, which relates to aspects of rides like g-forces that might induce nausea, and at the very top is route efficiency (i.e., getting to destinations as quickly as possible).
“Whether it’s the common scenario of being cut off on a freeway or one of the rare long tail events, an AV’s planner needs to correctly and consistently choose the right behavior,” continued the Level 5 team. “While there is no objective definition of the ‘right’ behavior, utilizing the AV hierarchy of needs and then applying a mix of systems to address these levels of needs helps disambiguate this complexity. Paired with a rigorous testing process, using this mixture of expert and learning-based systems has the potential to ultimately introduce a new benchmark for safety.”
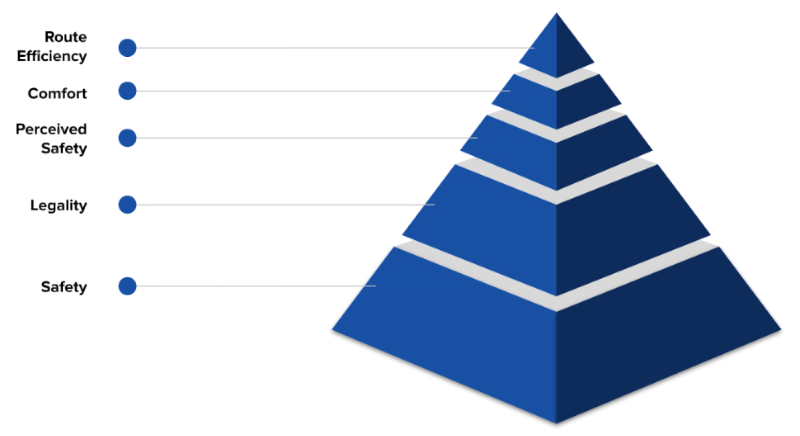
Above: A diagram of Lyft’s decision-making model.
Transparency about the systems underpinning driverless cars will undoubtedly be key in promoting public acceptance. According to a survey conducted by PSB Research and commissioned by Intel last year, only 21% of Americans would trade their cars for driverless vehicles and nearly half (43%) don’t feel safe around them. That’s despite the fact that about 94% of car crashes are caused by human error and that in 2016 the top three causes of traffic fatalities were distracted driving, drunk driving, and speeding.
A brief history of Lyft’s self-driving efforts
Lyft’s Level 5 team is a group of data scientists, applied researchers, product managers, operations managers, and others working to build a self-driving system for ride-sharing. Since the division was founded in July 2017, the group has developed novel 3D segmentation frameworks, new methods of evaluating energy efficiency in vehicles, and techniques for tracking vehicle movement using crowdsourced maps.
Earlier this year Lyft announced the opening of a new road test site in Palo Alto, California, near its Level 5 division’s headquarters. At the new center, engineers will mimic real-world driving scenarios involving intersections, traffic lights, roadway merges, pedestrian pathways, and other public road conditions, components of which will be reconfigurable.
The development comes after a year in which Lyft expanded access to its employee self-driving service in Palo Alto (where it has secured permission from city officials) with human safety drivers on board in a limited area. The company says in 2019 it increased the available routes “three-fold” and that it plans to grow the regions covered “rapidly.”
In November, Lyft revealed that it’s now driving four times more miles on a quarterly basis than it was six months ago and that it has about 400 employees dedicated to autonomous vehicle technology development globally (up from 300 previously). According to the company, 96% of people who try hailing a driverless vehicle in the Lyft app say they want to do so again.
In May, Lyft partnered with Google parent company Alphabet’s Waymo to enable customers to hail driverless Waymo cars from the Lyft app in Phoenix, and Lyft has an ongoing collaboration with self-driving car startup Aptiv, which makes a small fleet of autonomous vehicles available to Lyft customers in Las Vegas. More recently, Lyft released an open source data set for autonomous vehicle development it said was one of the largest of its kind, with over 55,000 human-labeled 3D annotated frames of traffic agents.