Watch all the Transform 2020 sessions on-demand here.
One of artificial intelligence’s most promising disciplines is computational photography — image processing that uses neural networks to correct artifacts, eliminate noise, and even generate works of art. During Re-Work‘s Deep Learning Summit in Boston, Massachusetts, Michael Sollami, a senior data scientist at Salesforce, presented a survey outlining the most significant computer graphics, digital photography, and computer vision developments in recent months, and the exciting new research on the horizon.
“[I think of] computer vision as a bag of tools to manipulate, enhance, combine, and synthesize,” Sollami told the audience members in attendance. “The most famous example is HDR, [which uses algorithms to] show high levels of detail.”
The first two studies in Sollami’s slide were from Nvidia. Both had to do with what’s called “hallucinated” photorealism, or the ability to generate photorealistic faces novelly, without a frame of reference beyond the data on which it has been trained.
June 5th: The AI Audit in NYC
Join us next week in NYC to engage with top executive leaders, delving into strategies for auditing AI models to ensure fairness, optimal performance, and ethical compliance across diverse organizations. Secure your attendance for this exclusive invite-only event.
The first, “Progressive Growing of GANs for Improved Quality, Stability, and Variation,” used a generative adversarial network, or GAN, to produce a photorealistic album of fake celebrities. (GANs comprise two neural networks: one that generates the images and a second network that checks the result, which the first neural network uses to improve its output.) The results aren’t perfect, but they’re convincing enough to give you pause.
The second of the two Nvidia studies, titled “AI Reconstructs Photos with Realistic Results,” used a neural network to fill in (“inpaint”) portions of an image that have been deleted or modified by creating masks and partial convolution predictions. Compared to conventional methods of filling in an image, it performs markedly better: There aren’t signs of blurred edges or granular degradation.
Next, Sollami moved onto the topic of “image to image translation,” also known as style transfer. It’s a category of machine learning popularized by apps like Prisma and Pikazo that uses deep learning to recompose an image in the style of another. “We have the ability … to do all of these magical things [at a high resolution],” Sollami said.
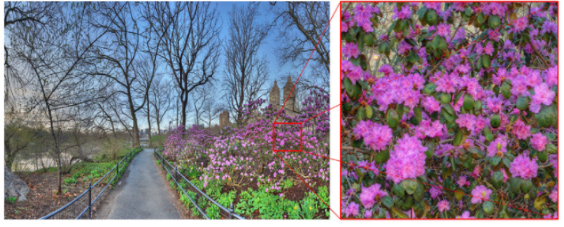
Above: Artwork from Daniel Ambrosi’s Dreamscapes collection.
He highlighted the work of Daniel Ambrosi, who worked with Google and Nvidia to produce landscapes inspired by 19th-century master paintings of the Hudson River School. Using custom modifications to Google’s DeepGram software, Ambrosi created large-format pictures with “unexpected” forms and content that can only be seen when viewed at a close distance. Each of the multi-hundred-megapixel panoramas took nine hours to produce.
“It’s really impressive work,” Sollami said. “When you step back and think about all the great things we can do with imaging, we’re basically rivaling human artists’ creativity and surpassing it.”
Another impressive example of neural network image enhancement came from researchers at the University of Illinois Urbana-Champaign, who trained a convolutional neural network to compare two sets of low-light photos: a grainier collection taken at short exposure and a crisper set taken at a longer exposure. The resulting system could optimize images from virtually any camera system in real time.
“This is an advancement in computational photography that’s going to make night photos you take a lot better,” Sollami said.
But AI-aided image enhancement has implications for other fields, too, such as biomedicine. Sollami mentioned a recent whitepaper from Intel that leveraged AI to classify and manipulate extremely high-resolution microscope images.
In the future, Sollami predicts that neural networks will become even more capable than they are today. “Everything’s going to be in super high resolution running in real time,” he said. Concretely, he predicts the dawn of “style transfer onto virtual worlds”: artificial neural nets that can generate digital environments, like those in a video game, from no more than a painting or picture.
“If you can hallucinate a Monet [with AI] in an instant, that makes it cheap,” he said. “But [I think of it as] a gut check. We have to rethink our intuitions about what’s creative and what is really art. These are just tools … you have computer-aided design, and this is just a higher version of that. The neural networks are just coming up with new palettes and textures.”