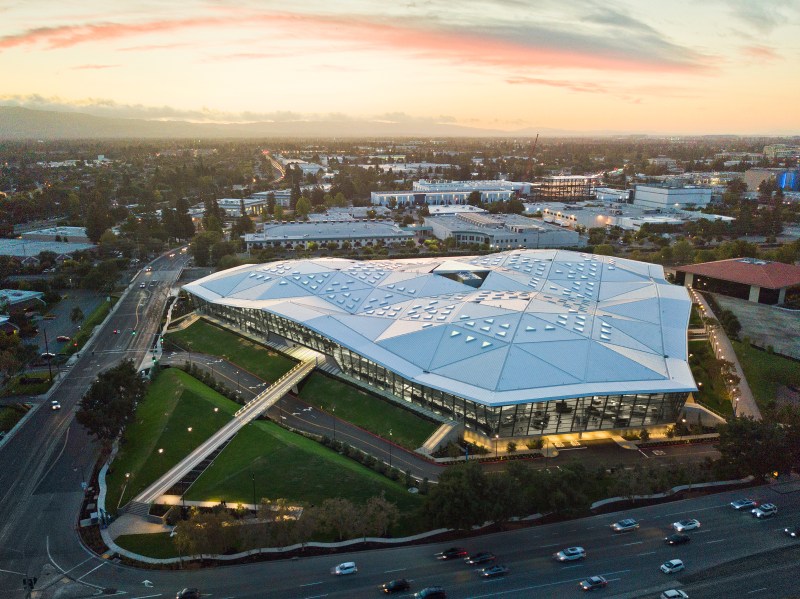
Above: Nvidia’s headquarters
Question: Can you tell us more about your partnership with Volkswagen?
Huang: There’s not much to go into. We’ve worked with them on a lot of projects. They’re a long-term partner of ours. It’s relatively well-known that we’re partnering with them on future products. There’s nothing to be announced right now, so I hesitate to say much more.
Question: With autonomous driving becoming a reality, do you have a next target as far as major areas for your company to move into?
Huang: I think you should come to GTC. [laughs] It’s in just a few months. We have some nice surprises for you.
June 5th: The AI Audit in NYC
Join us next week in NYC to engage with top executive leaders, delving into strategies for auditing AI models to ensure fairness, optimal performance, and ethical compliance across diverse organizations. Secure your attendance for this exclusive invite-only event.
Question: Can you talk more about how you’re training AI to work in autonomous vehicles?
Huang: Let me first explain how deep learning works. The benefit of deep learning is that it can have a great deal of diversity. Meaning, the way we teach our AI — we teach the AI examples of cars and examples of pedestrians and motorcycles and bicycles and trucks and signs. We give it positive examples. We also give it a lot of noisy examples. We also give it a lot of tortured examples. We change the shapes and sizes. We add a lot of noise. Then we try to fake it. We try to put all kinds of random things in to teach it to be more robust.
We’re trying to make the network as general as possible, as diverse as possible, and as robust as possible, just like humans. With our brains, we can see a fruit and say, “This isn’t exactly the same as every other fruit, but it’s also a fruit.” We generalize. The brain has a great deal of diversity. With many different types of fruits in many different environments, I can still recognize a fruit. Your brain is also robust. When I show you a plastic fruit, you’re not tricked into think it’s real. We have to teach AI to be robust, not to be easily confused.
The teaching of AI is an art in itself. Robustness, diversity, and generalization are all characteristics we’re trying to teach.
Question: One of the big surprises at the press conference on Sunday was support for adaptive sync. Can you elaborate on that decision and why you’re supporting it after competing against it so long?
Huang: We never competed against it. It was just never proven to work. As you know, we invented the whole area of adaptive sync. We invented the technology. As we were building G-Sync monitors, we were also calibrating and improving the performance of every panel maker and backlight maker and controller maker. We were lifting the whole industry. We could see that even the monitors that just generically went out the door were improving in quality.
Unfortunately, improving in quality does not make it work for adaptive sync, for G-Sync. In fact, we’ve been testing it for a long time. In all of the monitors we tested, the truth is that most of the FreeSync monitors do not work. They don’t even work with AMD’s graphics cards, because nobody tested it. We think that it’s a terrible idea to let a customer buy something believing in the promise of the product and then have it not work.
Our strategy is this: we will test every single card against every single monitor, against every single game. If it doesn’t work, we will say it doesn’t work. When it does work, we’ll automatically leverage it. Even when it doesn’t work, we’ll let people decide, “You know what, I’ll roll the dice.” But we believe that you have to test it to promise that it works. Unsurprisingly, most of them don’t work.
Question: Can you talk about the future of Shield and Tegra, especially now that Nintendo is using the Tegra in their Switch?
Huang: As you know, Shield TV is still unquestionably the best Android TV the world makes. We’ve updated the software now over 30 times. People are blown away by how much we continue to enhance it. There are more enhancements coming. We’re committed to it.
On mobile devices, we don’t think it’s necessary. We wouldn’t only build things to compete for share. If you’ve been watching us over the years, Nvidia is not a “Take someone else’s market share” company. I think that’s really angry. It’s an angry way to run a business. Creating new markets, expanding the horizon, creating things that the world doesn’t have, that’s a loving way to build a company. It inspires employees. They want to do that.
It’s really hard to get people — if you say, “What we are as a company, we’re going to build one just like theirs, but we’ll sell it cheaper,” it’s a weird sensibility. That’s not our style.
Question: Is there any possibility of a new Shield portable or tablet, then?
Huang: Only if the world needs it, or if the customer is underserved. At the moment I just don’t see it. When we first built it — I still have the original Shield portable. Every so often I pick it up and I think, “The world needs this.” But I don’t think so. Maybe not yet. I think Nintendo’s done such a great job. It’s hard to imagine doing that. I love what they did with it.
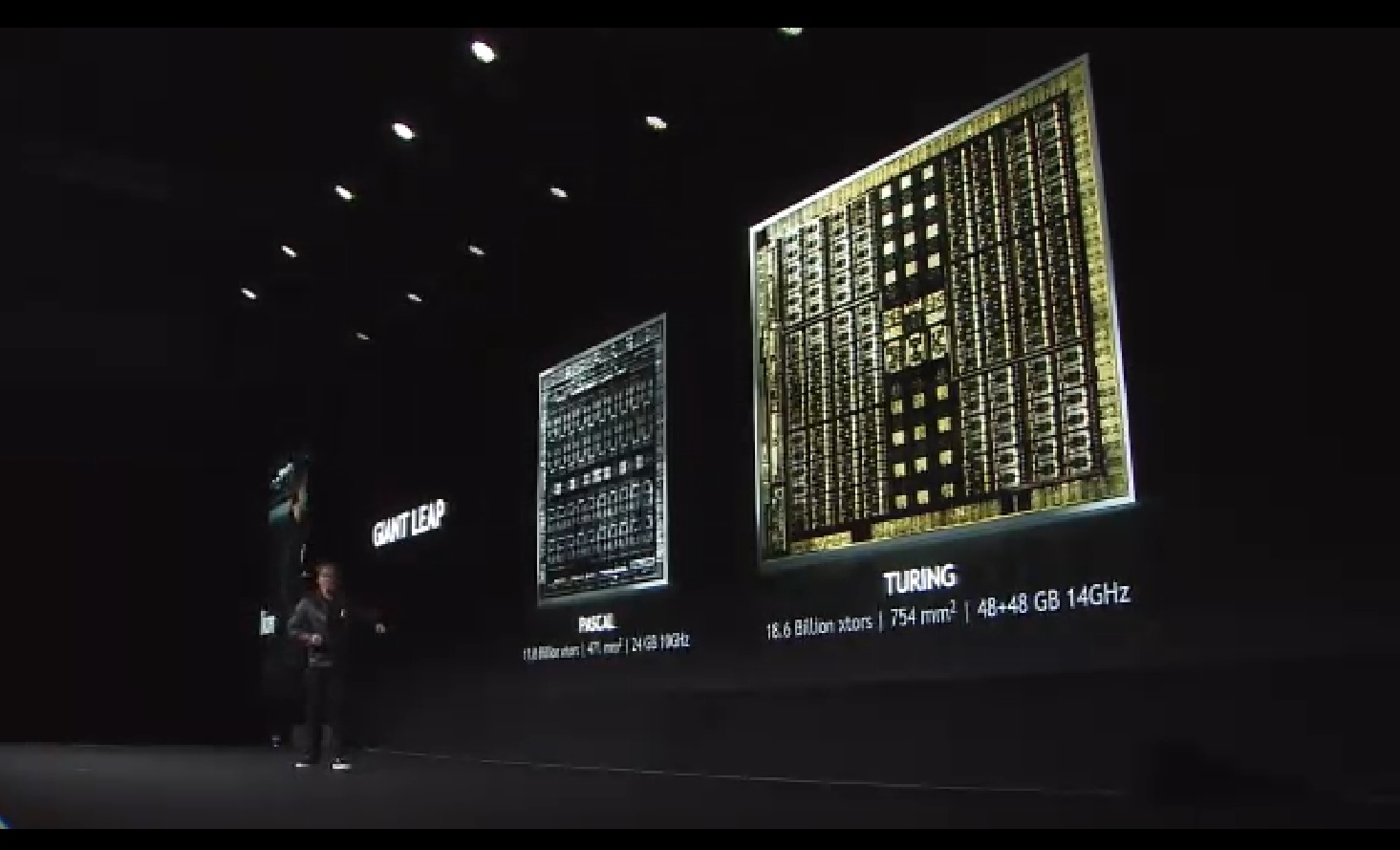
Above: Turing is 754 millimeters square.
Question: Can you talk a bit more generally about AI? It’s a big theme of this show, and it’s obviously embedded in RTX. What is the crossover like to other verticals for you, and what’s your general strategy for leadership in AI amongst chip companies?
Huang: As you know, we were the world’s first computing company to jump into and start building entire systems for deep learning, for AI. I believe that AI has three basic chapters. The first chapter is about creating the algorithms, the tools, and the computing systems for AI. The computing system we created for AI has a processor called V100, an interconnect called NVLink, a system called VGX, a domain-specific language called cuDNN, and it’s integrated into every framework in the world.
That entire stack, the full stack, is chapter one of deep learning. We’ve made it possible everywhere it’s available, every single cloud, every single computer maker in the world. We’ve democratized deep learning. Every company in the world can use it. We have customers in every country, every university, all over the place.
The second chapter is about deep learning being applied to services in the cloud. This is why the internet service companies went first, because they had the data. Google went first, and then Facebook and Amazon and Microsoft. All of the other people who have a lot of internet services — it could be LinkedIn or Netflix — they have lots of data, and they went second. The third phase is enterprise use. The health care industry, insurance industry, financial industry, transportation industry — all of those industries are now really red hot.
And then of course the next generation is where AI comes out of the cloud and into the physical world. It’s the robotics revolution. The first robots will be cars. The reason why is because the industry is large. We can make a difference. We can save lives. We can lower costs. We can make people’s lives better. It’s a very obvious use case. That’s where we’ll focus first.
The second is now manufacturing robots, service robots. The Amazon effect, people buying everything online and in the cloud, is causing delivery channels to change all over the world. There aren’t enough truck drivers, not by any stretch of the imagination. There’s no way to deliver a hamburger to a dorm room for millions of people. It’s just not possible. Now delivery and logistics robots are going to come out — small ones, medium-sized ones — delivering groceries and so on. The robot revolution, that autonomy, that’s the next chapter.
We’re involved in all of this. Our strategy here, from a hardware perspective, is our GPUs and DGX. Our strategy here is all of our cloud systems that we offer to our cloud customers. We call them HGX, for hyperscale. Next is all of our enterprise, all of our partners there. Next is our Drive system and our robotics system, Isaac. We’re just methodically going through building out the future of AI as the technology evolves.
Question: Can you tell us more about your problem with excess inventory and how that will be resolved?
Huang: It’s completely a crypto hangover issue. Remember, we basically shipped no new GPU in the market, to the channel, for one quarter. But the amount of excess inventory and market demand, channel velocity — you just have inventory divided by velocity, and that’s time. We said that it would take one to two quarters for all the channel inventory to sell out. 1080Ti has sold out. 1080 has sold out. 1070 has sold out. 1070Ti has sold out. In several more weeks 1060s will sell out. Then we can go back to business.
I think the world would be very sad if Nvidia never shipped another GPU. That would be very bad.